in Danish
AI and Machine Learning is what’s everybody is talking about in 2017. At eCapacity, we want to turn the talk into actions. From buzzwords to business.
Take a look at this case story we did with Codan, where we are using AI to predict and personalize the most relevant homepage for you – out of 60 different versions of the homepage and creating a huge uplift in conversion.
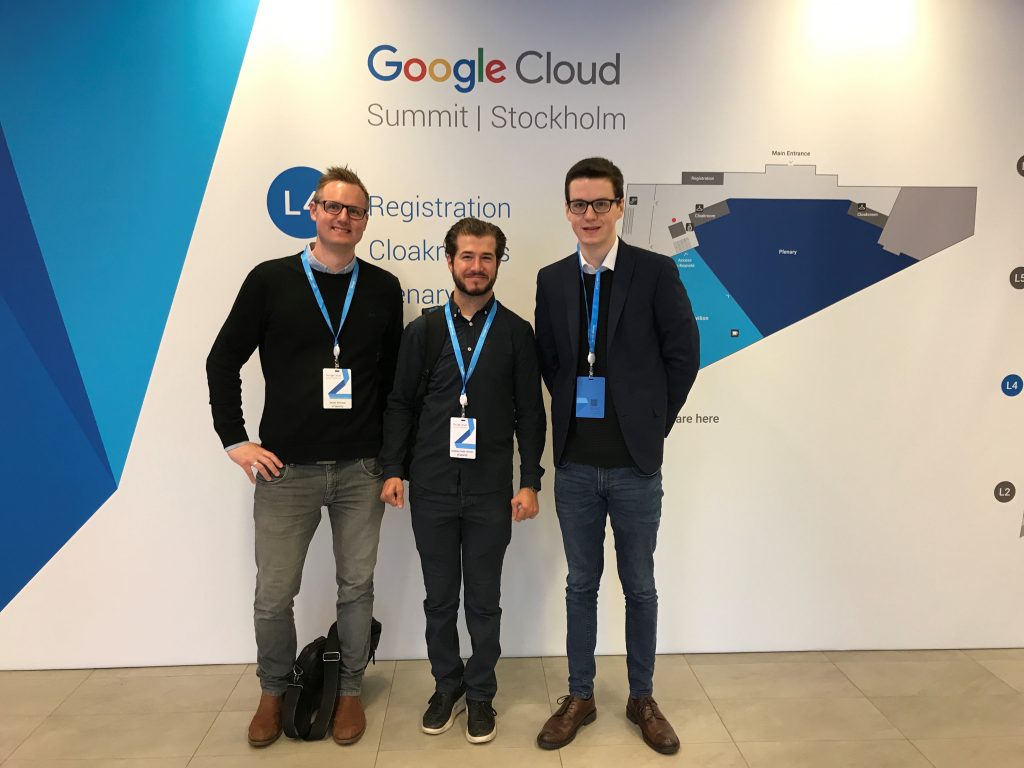
We are always on the lookout for inspiration on practical use cases for Machine Learning to turn data into business value.
Last week we went to Stockholm, to attend the Google Cloud Summit, with focus on AI and Machine Learning and here is five inspiring use cases for Machine Learning.
MQT: Predicting high value start-up investments
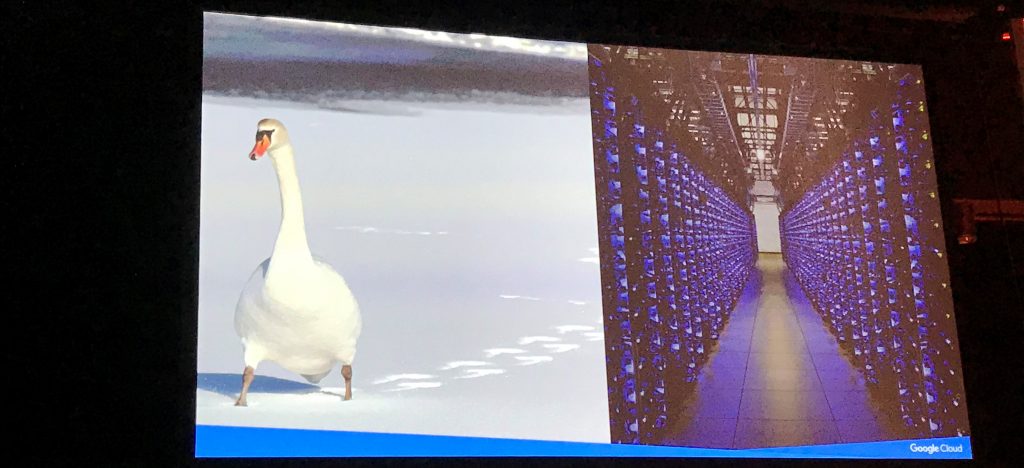
MQT combines the vast amount of digital footprint with computer power and machine learning to predict what start-ups will become highly valuable. 15,000 new companies are launched every day, so how do you know which ones to bet on?
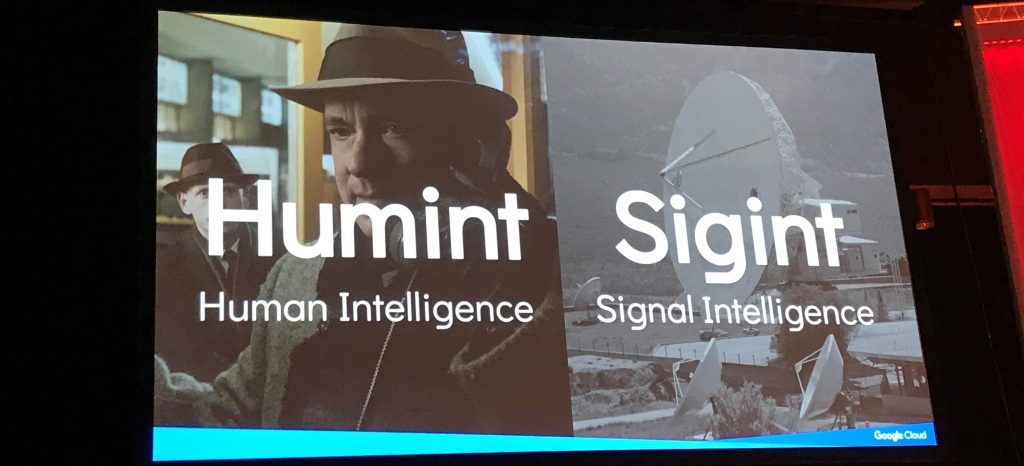
MQT uses machine learning and human judgement to identify the common patterns and characteristics of successful start-ups so they can invest in them before everyone else.
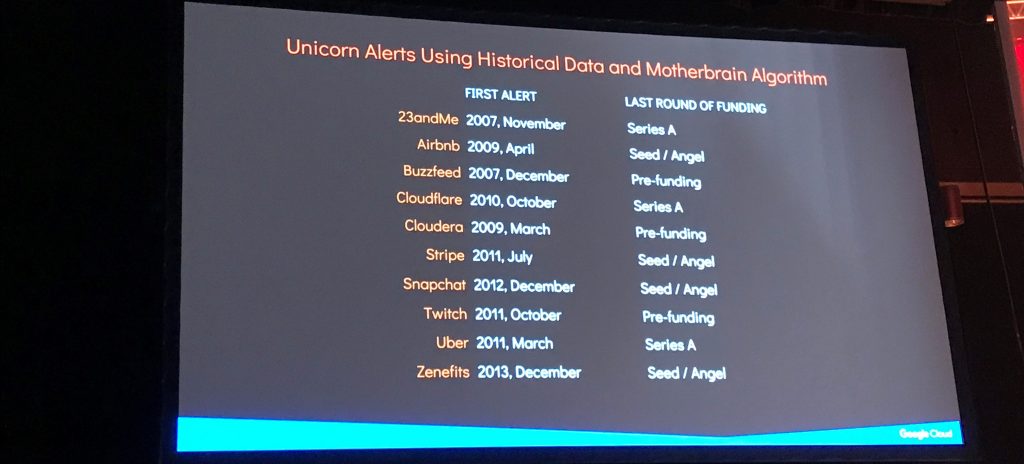
Aker BP – predicting maintenance jobs on an oil rig
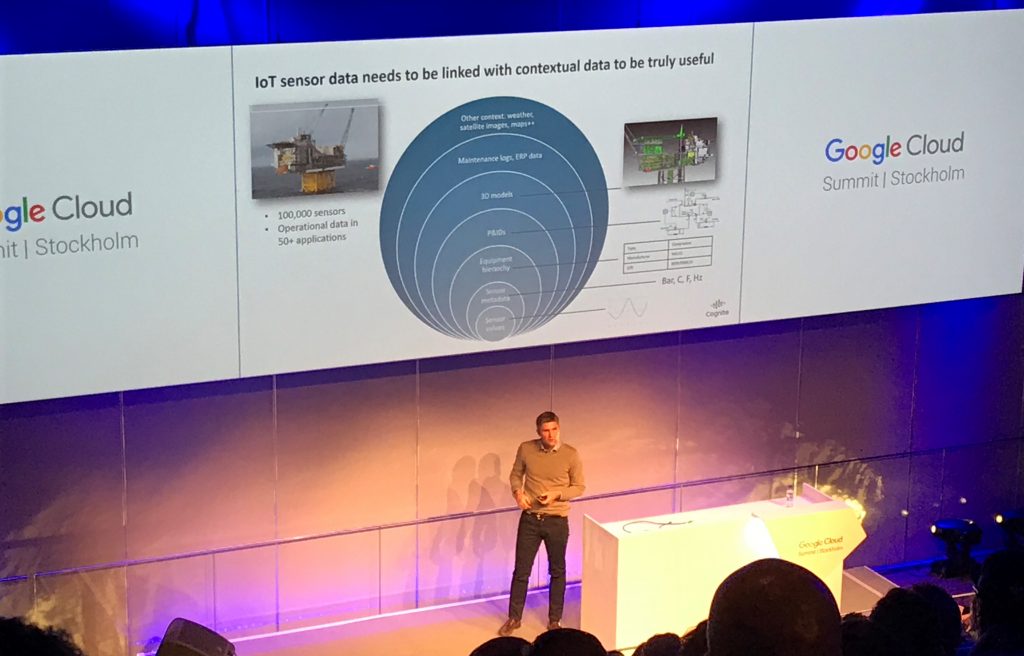
The oil industry is losing market shares and needs to be more efficient than ever to survive.
Aker BP has over 100,000 sensors on its new oil rig, called Ivar Aasen, and they are using machine learning to find patterns in the data across more than 50 applications.
Maintenance on a compressor (turbine) can cost as much as 30 million EUR and by using predictive analytics they have decreased maintenance from every 3 years to every 6 years and saved a lot of money.
Deli ice cream: Optimizing ice cream delivery in Singapore’s traffic nightmare
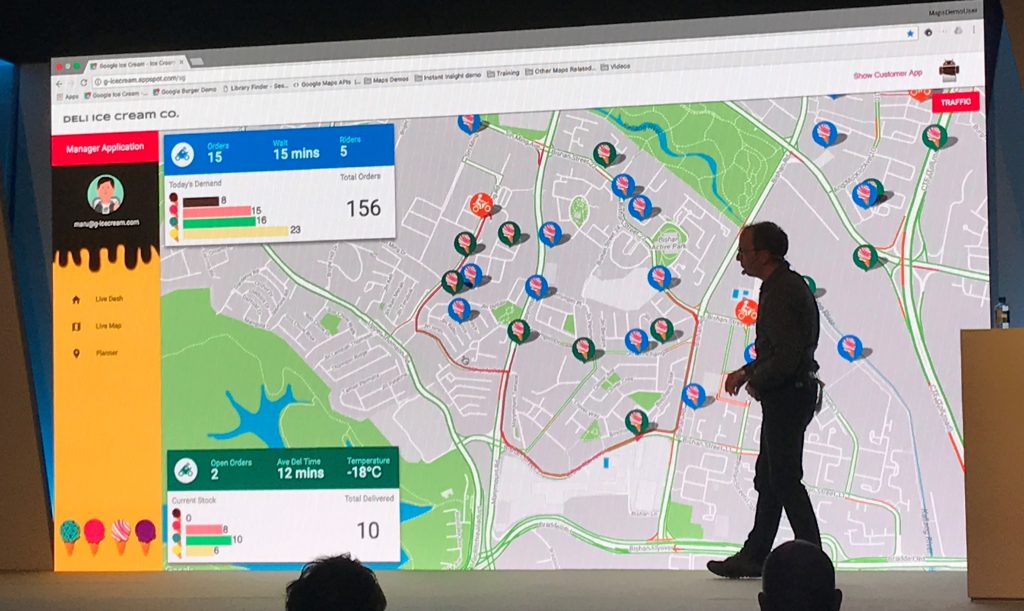
Google used the fictional company Deli Ice Cream, to demo the machine learning capabilities of Google Maps.
Deli ice cream is using bikes to deliver ice creams in the heart of Singapore. The bikes are fitted with a freezer to keep the ice cream cold – but only for a limited time.
Therefore, Deli ice cream uses the Google Maps API to optimize delivery times.
The system combines the location and current ice cream stock of the bikes, with machine learning and the current traffic situation, to predict which bike can get to the customer fastest. The fastest route is sent to the bike and the estimated time of arrival is sent to the user.
Instant insights: Real-time predicted relevant ads for customers in taxis
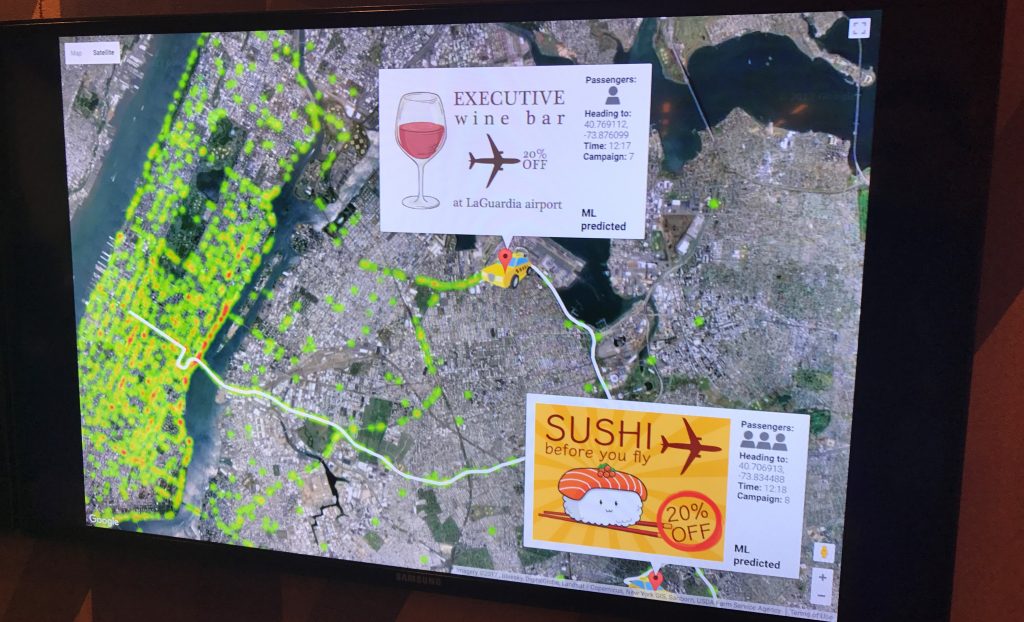
Another fictional machine learning demo by Google was Instant Insights.
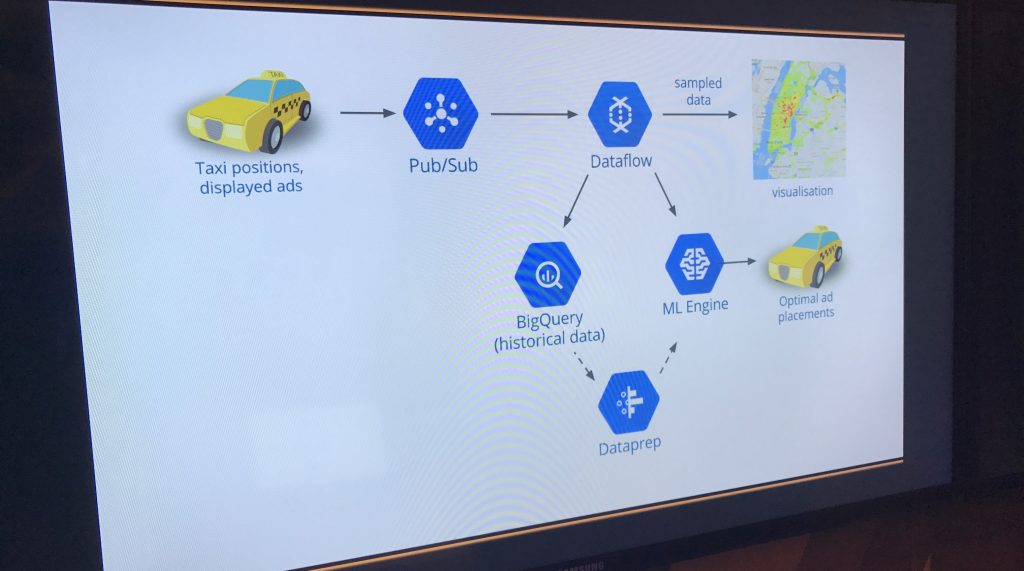
By using machine learning and real-time data from taxi trips like start location, number of passengers, time of day and the route of the taxi to predict where the taxi is going and present relevant ads for restaurants and shops near the destination.
The user can click on the ads to find more information and using reinforcement learning, the system can use this information to learn which ads are most relevant to the user and improve the system.
Spotify – Real-time streaming insights to artists
Okay, this one is not about Machine Learning, but it does involve a lot of data, which always make us turn our heads. And hey! Five is a better number for a headline than four, right?
Now, we are not saying that Spotify has a lot of data – but not a lot of companies has a 2500-node Hadoop cluster.

To turn data into insights faster, Spotify changed their Hadoop cluster for a Google BigQuery solution, which allows them to not only give data insights to it’s 740 active users on BigQuery (that’s 25% of the employees at Spotify!) but also to give the artist access to real-time streaming statistics for their songs.
Okay, you want that fifth use case for machine learning?
You got it: Google Search.
Almost 9 years ago, Google search started moving from man-made rules to computer made rules, by defining what a good search result looks like, and then let the computer figure out what the rules should be.
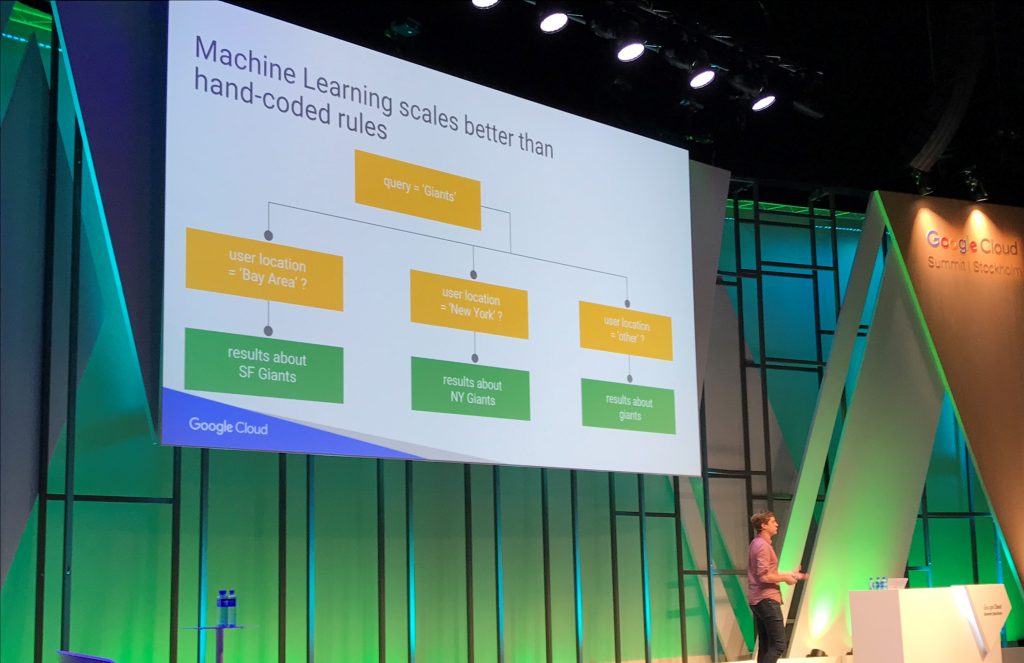
Google had more than 3 million man-made rules to figure out that best search result for queries like “Giants” based on whether your location was in New York or San Francisco.
3 million man-made rules!
By replacing those rules with a criteria for a good search result, like high click-through-rate, the machine looks at your location and all other information it knows about you, to test its rules and improve the search results automatically.
in Danish